Contrastive Predictive Coding¶
Contrastive Predictive Coding, CPC, is an autoregressive model combined with InfoNCE loss1.
Predictive Coding
As a related topic, predictive coding is a different scheme than backpropagation. Predictive coding updates the weights using local updating rules only2.
There are two key ideas in CPC:
- Autoregressive models in latent space, and
- InfoNCE loss that combines mutual information and NCE.
For the series of segments, \(\{x_t\}\), we apply an encoder on each segment, and calculate the latent space, \(\{{\color{blue}\hat x_t}\}\). The latent space \(\{{\color{blue}\hat x_t}\}\) is then modeled using an autoregressive model to calculate the coding, \(\{{\color{red}c_t}\}\).
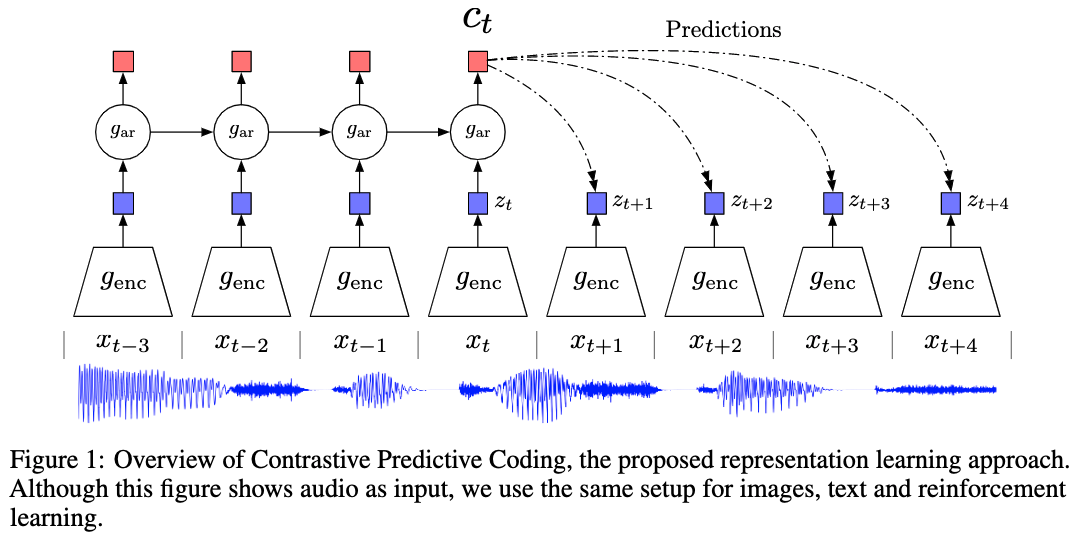
The loss is built on NCE to estimate the lower bound of mutual information,
where \(f_k(x_{x+i}, c_t)\) is estimated using a log-bilinear model, \(f_k(x_{x+i}, c_t) = \exp\left( z_{t+i} W_i c_t \right)\). This is also a cross entropy loss.
Minimizing \(\mathcal L\) leads to a \(f_k\) that estimates the ratio1
We can perform downstream tasks such as classifications using the encoders.
Maximizing this lower bound?
This so-called lower bound for mutual information in this case is not always going to work[^Newell2020]. In some cases, the representations learned using this lower bound doesn't help or even worsen the performance of downstream tasks.
Code¶
-
van den Oord A, Li Y, Vinyals O. Representation learning with Contrastive Predictive Coding. arXiv [cs.LG]. 2018. Available: http://arxiv.org/abs/1807.03748 ↩↩
-
Millidge B, Tschantz A, Buckley CL. Predictive coding approximates backprop along arbitrary computation graphs. 2020.http://arxiv.org/abs/2006.04182. ↩